Documents
Poster
Gluformer: Transformer-Based Personalized Glucose Forecasting with Uncertainty Quantification
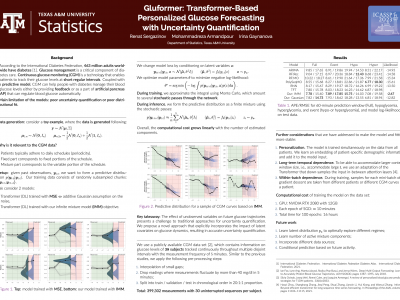
- Citation Author(s):
- Submitted by:
- Renat Sergazinov
- Last updated:
- 21 May 2023 - 2:32am
- Document Type:
- Poster
- Document Year:
- 2023
- Event:
- Presenters:
- Renat Sergazinov
- Paper Code:
- 162
- Categories:
- Log in to post comments
Deep learning models achieve state-of-the art results in predicting blood glucose trajectories, with a wide range of architectures being proposed. However, the adaptation of such models in clinical practice is slow, largely due to the lack of uncertainty quantification of provided predictions. In this work, we propose to model the future glucose trajectory conditioned on the past as an infinite mixture of basis distributions (i.e., Gaussian, Laplace, etc.). This change allows us to learn the uncertainty and predict more accurately in the cases when the trajectory has a heterogeneous or multi-modal distribution. To estimate the parameters of the predictive distribution, we utilize the Transformer architecture. We empirically demonstrate the superiority of our method over existing state-of-the-art techniques both in terms of accuracy and uncertainty on the synthetic and benchmark glucose data sets.