Documents
Poster
Gumbel-NeRF: Representing Unseen Objects as Part-Compositional Neural Radiance Fields
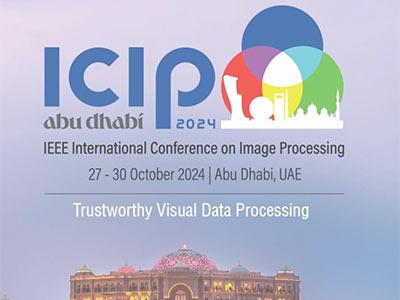
- Citation Author(s):
- Submitted by:
- Ikuro Sato
- Last updated:
- 8 November 2024 - 8:45pm
- Document Type:
- Poster
- Document Year:
- 2024
- Event:
- Presenters:
- Ikuro Sato
- Paper Code:
- 1453
- Categories:
- Log in to post comments
We propose Gumbel-NeRF, a mixture-of-expert (MoE) neural radiance fields (NeRF) model with a hindsight expert selection mechanism for synthesizing novel views of unseen objects. Previous studies have shown that the MoE structure provides high-quality representations of a given large-scale scene consisting of many objects. However, we observe that such a MoE NeRF model often produces low-quality representations in the vicinity of experts’ boundaries when applied to the task of novel view synthesis of an unseen object from one/few-shot input. We find that this deterioration is primarily caused by the foresight expert selection mechanism, which may leave unnatural discontinuity in the object shape near the experts’ boundaries. Gumbel-NeRF adopts a hindsight expert selection mechanism, which guarantees continuity in the density field even near the experts’ boundaries. Experiments using the SRN cars dataset demonstrate the superiority of Gumbel-NeRF over the baselines in terms of various image quality metrics.