Documents
Presentation Slides
Hierarchy-aware Loss Function on a Tree Structured Label Space for Audio Event Detection
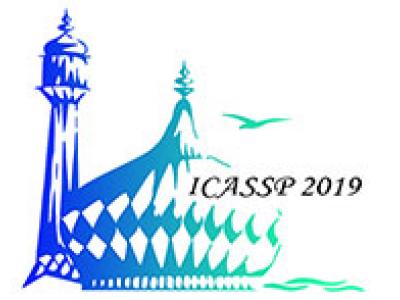
- Citation Author(s):
- Submitted by:
- Arindam Jati
- Last updated:
- 10 May 2019 - 1:02am
- Document Type:
- Presentation Slides
- Document Year:
- 2019
- Event:
- Presenters:
- Jaekwon Yoo
- Paper Code:
- 2594
- Categories:
- Log in to post comments
The paper introduces a hierarchy-aware loss function in a Deep Neural Network for an audio event detection task that has a bi-level tree structured label space. The goal is not only to improve audio event detection performance at all levels in the label hierarchy, but also to produce better audio embeddings. We exploit the label tree structure to preserve that information in the hierarchy-aware loss function. Two different loss functions are separately employed. First, a triplet loss with probabilistic multi-level batch mining is introduced. Second, a quadruplet learning method is applied, which is a special case of generalized triplet learning for bi-level label taxonomy. The training is performed in a multi-task learning framework by jointly optimizing cross entropy based loss and hierarchy-aware loss function. The proposed method is found to outperform the baseline cross entropy based models at both levels of the hierarchy. The multi-task model is also able to learn better audio representations as observed in our clustering experiments. Moreover, the model is shown to transfer well when an out-of-domain dataset is used for evaluation.
https://ieeexplore.ieee.org/document/8682341