Documents
Poster
High Resolution Water Segmentation for Autonomous Unmanned Surface Vehicles: A Novel Dataset and Evaluation
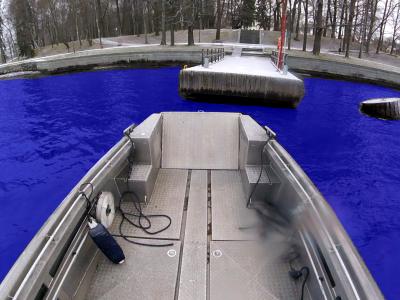
- Citation Author(s):
- Submitted by:
- Jussi Taipalmaa
- Last updated:
- 24 October 2019 - 2:10pm
- Document Type:
- Poster
- Document Year:
- 2019
- Event:
- Presenters:
- Jussi Taipalmaa
- Categories:
- Log in to post comments
Even though Unmanned Surface Vehicles (USVs) are increasingly used to perform various laborious and expensive off-shore tasks, they still require an extensive dedicated crew supporting and ensuring the safety of their operations. The recent developments in computer vision and robotics further fueled the interest on developing \textit{autonomous} USVs that will overcome the aforementioned limitations, unleashing their full potential. One of the most vital and fundamental tasks in order to automate and ensure the safety of USV operations is to perform water segmentation. Despite the importance of developing such segmentation methods, there is a lack of high resolution publicly available datasets, which are suitable for training and evaluating deep learning methods. The main contribution of this paper is collecting, annotating and releasing a publicly available high-resolution dataset for developing deep learning algorithms for water segmentation in a Nordic lake environment. Furthermore, we adapt a deep learning algorithm previously applied for road segmentation to the water segmentation task. While the algorithm obtains a high accuracy, the results also allow for identifying critical limitations of the approach. Finally, we propose and evaluate a novel lightweight fully convolutional neural network architecture, fully adapted to the needs of water segmentation from high resolution images.