Documents
Poster
HYBRID ATTENTION-BASED PROTOTYPICAL NETWORKS FOR FEW-SHOT SOUND CLASSIFICATION
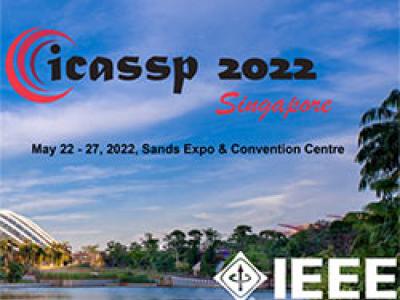
- Citation Author(s):
- Submitted by:
- You Wang
- Last updated:
- 14 May 2022 - 4:36pm
- Document Type:
- Poster
- Document Year:
- 2022
- Event:
- Presenters:
- You Wang
- Paper Code:
- AUD-21.5
- Categories:
- Log in to post comments
In recent years, prototypical networks have been widely used
in many few-shot learning scenarios. However, as a metric-
based learning method, their performance often degrades in
the presence of bad or noisy embedded features, and outliers
in support instances. In this paper, we introduce a hybrid at-
tention module and combine it with prototypical networks for
few-shot sound classification. This hybrid attention module
consists of two blocks: a feature-level attention block, and
an instance-level attention block. These two attention mech-
anisms can highlight key embedded features and emphasize
crucial support instances respectively. The performance of
our model was evaluated using the ESC-50 dataset and the
noiseESC-50 dataset. The model was trained in a 10-way
5-shot scenario and tested in four few-shot cases, namely 5-
way 1-shot, 5-way 5-shot, 10-way 1-shot, and 10-way 5-shot.
The results demonstrate that by adding the hybrid attention
module, our model outperforms the baseline prototypical net-
works in all four scenarios.