Documents
Poster
ICASSP 2019 Poster - Privacy-preserving Paralinguistic Tasks
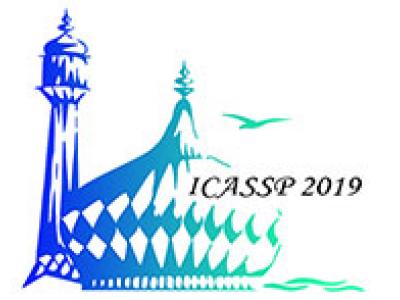
- Citation Author(s):
- Submitted by:
- Francisco Teixeira
- Last updated:
- 10 May 2019 - 10:21am
- Document Type:
- Poster
- Document Year:
- 2019
- Event:
- Presenters:
- Francisco Teixeira
- Paper Code:
- 3713
- Categories:
- Log in to post comments
Speech is one of the primary means of communication for humans. It can be viewed as a carrier for information on several levels as it conveys not only the meaning and intention predetermined by a speaker, but also paralinguistic and extralinguistic information about the speaker’s age, gender, personality, emotional state, health state and affect. This makes it a particularly sensitive biometric, that should be protected. In this work we intent to explore how Leveled Homomorphic Encryption can be combined with a Neural Network to create a privacy-preserving machine learning framework for speech-based health-related tasks. In particular, we will apply this framework to the detection and assessment of a Cold, Depression and Parkinson’s Disease. Moreover, we will show how using a Quantized Neural Network, with discretized weights, allows us to apply a Leveled Homomorphic Encryption technique called batching that can be utilized to reduce the effective computational cost of this framework.