Documents
Poster
Image Super-Resolution using CNN Optimised by Self-Feature Loss
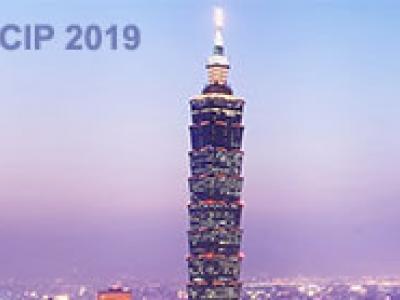
- Citation Author(s):
- Submitted by:
- ZHAO GAO
- Last updated:
- 19 September 2019 - 10:54am
- Document Type:
- Poster
- Document Year:
- 2019
- Event:
- Presenters:
- Zhao Gao
- Paper Code:
- 1030
- Categories:
- Log in to post comments
Despite the success of state-of-the-art single image superresolution algorithms using deep convolutional neural networks in terms of both reconstruction accuracy and speed of execution, most proposed models rely on minimizing the mean square reconstruction error. More recently, inspired by transfer learning, Mean Square Error (MSE)-based content loss estimation has been replaced with loss calculated on feature maps of the pre-trained networks, e.g. VGG-net used for ImageNet classification. We demonstrate that this alternative approach is sub-optimal and adds false colour and mosaicking artefacts in the reconstructed images. In this paper, we present the first Convolutional Neural Network (CNN) capable of optimizing its parameters by minimizing the loss of in-network, self-features. To achieve this, we propose a new loss function for a light CNN architecture, which contains a mapping of residual blocks between the low and high resolution images. Our proposed method performs better than existing methods that apply perceptual loss by effectively suppressing false colour-effects. We show that the in-network features used for the determination of the loss function will give new insights for future research and applications when designing deep learning networks for other computer vision tasks such as demosaicing and denoising.