Documents
Presentation Slides
Importance Sampling Based Unsupervised Federated Representation Learning
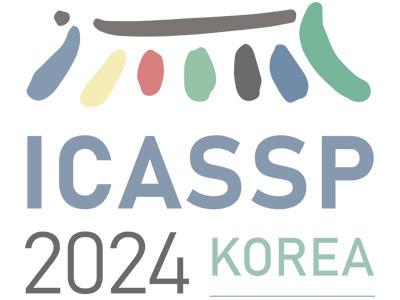
- DOI:
- 10.60864/089j-qh18
- Citation Author(s):
- Submitted by:
- Nazreen Ambalath
- Last updated:
- 6 June 2024 - 10:23am
- Document Type:
- Presentation Slides
- Document Year:
- 2024
- Event:
- Presenters:
- Nazreen Shah Ambalath
- Paper Code:
- MLSP-L14.5
- Categories:
- Keywords:
- Log in to post comments
The use of AI has led to the era of pervasive intelligence, marked by a proliferation of smart devices in our daily lives. Federated Learning (FL) enables machine learning at the edge without having to share user-specific private data with an untrusted third party. Conventional FL techniques are supervised learning methods, where a fundamental challenge is to ensure that data is reliably annotated at the edge. Another approach is to obtain rich and informative representations ofunlabeled data, which is suitable for downstream tasks. We propose a novel IS-FedVAE framework where we use importance sampling to federate a global VAE framework, allowing us to learn the global latent space distribution using local latent space distributions at the edge. We evaluate the representation in a stand-alone manner using linear probe, where we freeze the backbone representation and measure the accuracy of a downstream classifier. Furthermore, we demonstrate that IS-FedVAE outperforms state-of-the-art unsupervised FL learning baselines. We also show that IS-FedVAE is insensitive to varying levels of heterogeneity, scalable to varying numbers of clients, and robust to the changing number of local epochs.