Documents
Presentation Slides
IMPROVED META LEARNING FOR LOW RESOURCE SPEECH RECOGNITION
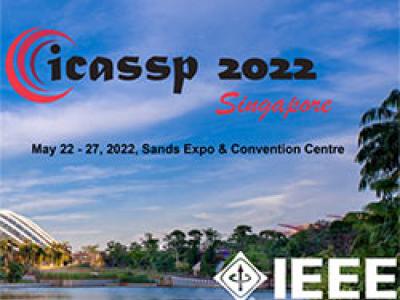
- Citation Author(s):
- Submitted by:
- Satwinder singh
- Last updated:
- 13 May 2022 - 10:50pm
- Document Type:
- Presentation Slides
- Document Year:
- 2022
- Event:
- Presenters:
- Satwinder Singh
- Paper Code:
- 5826
- Categories:
- Log in to post comments
We propose a new meta learning based framework for low resource speech recognition that improves the previous model agnostic meta learning (MAML) approach. The MAML is a simple yet powerful meta learning approach. However, the MAML presents some core deficiencies such as training instabilities and slower convergence speed. To address these issues, we adopt multi-step loss (MSL). The MSL aims to calculate losses at every step of the inner loop of MAML and then combines them with a weighted importance vector. The importance vector ensures that the loss at the last step has more importance than the previous steps. Our empirical evaluation shows that MSL significantly improves the stability of the training procedure and it thus also improves the accuracy of the overall system. Our proposed system outperforms MAML based low resource ASR system on various languages in terms of character error rates and stable training behavior.