Documents
Poster
Improving Cross-domain Few-shot Classification with Multilayer Perceptron
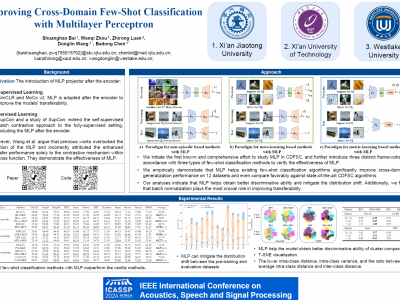
- DOI:
- 10.60864/5yr0-ys63
- Citation Author(s):
- Submitted by:
- Shuanghao Bai
- Last updated:
- 29 March 2024 - 10:33pm
- Document Type:
- Poster
- Document Year:
- 2024
- Event:
- Presenters:
- Shuanghao Bai
- Paper Code:
- MLSP-P3.4
- Categories:
- Log in to post comments
Cross-domain few-shot classification (CDFSC) is a challenging and tough task due to the significant distribution discrepancies across different domains. To address this challenge, many approaches aim to learn transferable representations. Multilayer perceptron (MLP) has shown its capability to learn transferable representations in various downstream tasks, such as unsupervised image classification and supervised concept generalization. However, its potential in the few-shot settings has yet to be comprehensively explored. In this study, we investigate the potential of MLP to assist in addressing the challenges of CDFSC. Specifically, we introduce three distinct frameworks incorporating MLP in accordance with three types of few-shot classification methods to verify the effectiveness of MLP. We reveal that MLP can significantly enhance discriminative capabilities and alleviate distribution shifts, which can be supported by our expensive experiments involving 10 baseline models and 12 benchmark datasets. Furthermore, our method even compares favorably against other state-of-the-art CDFSC algorithms.