Documents
Poster
Incorporating Intra-Spectral Dependencies With A Recurrent Output Layer For Improved Speech Enhancement
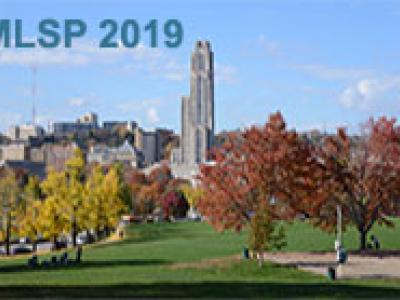
- Citation Author(s):
- Submitted by:
- KHANDOKAR MD NAYEM
- Last updated:
- 13 October 2019 - 1:29pm
- Document Type:
- Poster
- Document Year:
- 2019
- Event:
- Presenters:
- Khandokar Md. Nayem
- Paper Code:
- 143
- Categories:
- Log in to post comments
Deep-learning based speech enhancement systems have offered tremendous gains, where the best performing approaches use long short-term memory (LSTM) recurrent neural networks (RNNs) to model temporal speech correlations. These models, however, do not consider the frequency-level correlations within a single time frame, as spectral dependencies along the frequency axis are often ignored. This results in inaccurate frequency responses that negatively affect perceptual quality and intelligibility. We propose a deep-learning approach that considers temporal and frequency-level dependencies. More specifically, we enforce spectral-level dependencies within each spectral time frame through the introduction of a recurrent output layer that models the Markovian assumption along the frequency axis. We evaluate our approach in a variety of speech and noise environments, and objectively show that this recurrent spectral layer offers performance gains over traditional approaches. We also show that our approach outperforms recent approaches that consider frequency-level dependencies.