Documents
Presentation Slides
Independent Vector Analysis using Semi-Parametric Density Estimation via Multivariate Entropy Maximization
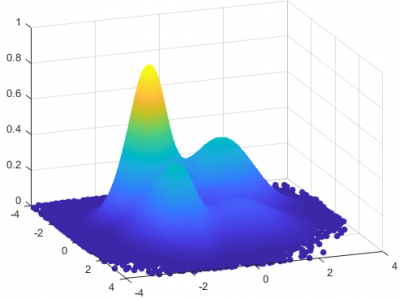
- Citation Author(s):
- Submitted by:
- Lucas Damasceno
- Last updated:
- 17 June 2021 - 12:09pm
- Document Type:
- Presentation Slides
- Document Year:
- 2021
- Event:
- Presenters:
- Lucas Damasceno
- Paper Code:
- MLSP-35.1
- Categories:
- Log in to post comments
Due to the wide use of multi-sensor technology, analysis of multiple sets of data is at the heart of many challenging engineering problems. Independent vector analysis (IVA), a recent generalization of independent component analysis (ICA), enables the joint analysis of datasets and extraction of latent sources through the use of a simple yet effective generative model. However, the success of IVA is tied to proper estimation of the probability density function (PDF) of the multivariate latent sources; information that is generally unknown. In this work, we propose a new flexible and efficient multivariate PDF estimation technique based on the maximum entropy principle and apply this technique to the development of an effective IVA algorithm that successfully matches multivariate latent sources from a wide range of distributions. We verify the effectiveness of the new estimation technique and further demonstrate the superior performance of the new IVA algorithm numerically using simulated data.
Comments
zoisboukouvalas.github.io/
zoisboukouvalas.github.io/