Documents
Presentation Slides
Insights into the behaviour of multi-task deep neural networks for medical image segmentation
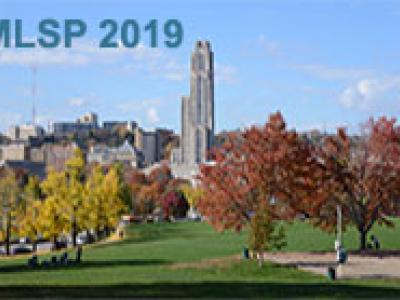
- Citation Author(s):
- Submitted by:
- Lukasz Bienias
- Last updated:
- 1 November 2019 - 11:34am
- Document Type:
- Presentation Slides
- Document Year:
- 2019
- Event:
- Presenters:
- Lukasz Tomasz Bienias
- Categories:
- Log in to post comments
Glandular morphology is used by pathologists to assess the malignancy of different adenocarcinomas. This process involves conducting gland segmentation task. The common approach in specialised domains, such as medical imaging, is to design complex architectures in a multi-task learning setup. Generally, these approaches rely on substantial postprocessing efforts. Moreover, a predominant notion is that general purpose models are not suitable for gland instance segmentation. We analyse the behaviour of two architectures: SA-FCN and Mask R-CNN. We compare the impact of post-processing on the final predictive results and the performance of generic and specific models for the gland segmentation problem. Our results highlight the dependency of post-processing on tailored models as well as comparable results when using a generic model. Thus, in the interest of time, it is worth considering to use and improve generic models as opposed to design complex architectures when tackling new domains.