Documents
Presentation Slides
Iterative Autoregressive Generation for Abstractive Summarization
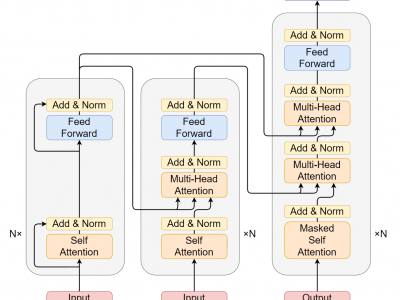
- DOI:
- 10.60864/xzb0-tt62
- Citation Author(s):
- Submitted by:
- Jiaxin Duan
- Last updated:
- 6 June 2024 - 10:54am
- Document Type:
- Presentation Slides
- Document Year:
- 2024
- Event:
- Presenters:
- Jiaxin Duan
- Paper Code:
- 5396
- Categories:
- Keywords:
- Log in to post comments
Abstractive summarization suffers from exposure bias caused by the teacher-forced maximum likelihood estimation (MLE) learning, that an autoregressive language model predicts the next token distribution conditioned on the exact pre-context during training while on its own predictions at inference. Preceding resolutions for this problem straightforwardly augment the pure token-level MLE with summary-level objectives. Although this method effectively exposes a model to its prediction errors during summary-level learning, such errors accumulate in the unidirectional autoregressive generation and further limit the learning efficiency. To address this problem, we imitate the human behavior of revising a manuscript multiple times after writing it and introduce a novel iterative autoregressive summarization (IARSum) paradigm, which iteratively rewrites a generated summary to approximate an errorless version. Concretely, IARSum performs iterative revisions after summarization, where the output of the previous revision is taken as the input for the next, and a minimum-risk training strategy is used to ensure that the original summary is effectively polished in every revision round. We conduct extensive experiments on two widely used datasets and show the new or matched state-of-the-art performance of IARSum.
Comments
This paper introduces a
This paper introduces a revision-based multi-iteration method for the training of summarization models. Reviewers in general are positive about the idea of the paper and the results seem promising. There are some concerns regarding the suitability of the paper to ICASSP as the paper is an NLP paper. I see no issue with this as the paper was submitted to the “Speech and Language Processing” track.