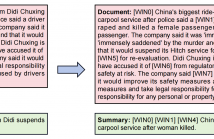
- Read more about Alleviating Hallucinations via Supportive Window Indexing in Abstractive Summarization
- 1 comment
- Log in to post comments
Abstractive summarization models learned with maximum likelihood estimation (MLE) have been proven to produce hallucinatory content, which heavily limits their real-world
applicability. Preceding studies attribute this problem to the semantic insensitivity of MLE, and they compensate for it with additional unsupervised learning objectives that maximize the metrics of document-summary inferring, however, resulting in unstable and expensive model training. In this paper, we propose a novel supportive windows indexing
- Categories:
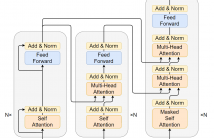
- Read more about Iterative Autoregressive Generation for Abstractive Summarization
- 1 comment
- Log in to post comments
Abstractive summarization suffers from exposure bias caused by the teacher-forced maximum likelihood estimation (MLE) learning, that an autoregressive language model predicts the next token distribution conditioned on the exact pre-context during training while on its own predictions at inference. Preceding resolutions for this problem straightforwardly augment the pure token-level MLE with summary-level objectives.
- Categories: