Documents
Presentation Slides
Joint Signal Recovery and Graph Learning from Incomplete Time-Series
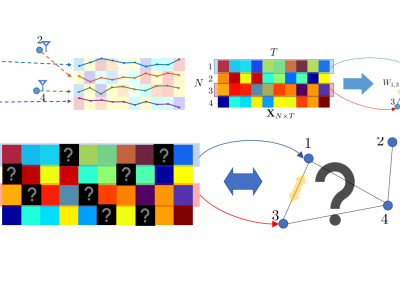
- DOI:
- 10.60864/5dsp-w521
- Citation Author(s):
- Submitted by:
- Amirhossein Javaheri
- Last updated:
- 6 June 2024 - 10:24am
- Document Type:
- Presentation Slides
- Document Year:
- 2024
- Event:
- Presenters:
- Daniel P. Palomar
- Paper Code:
- SS-L6.5
- Categories:
- Log in to post comments
Learning a graph from data is the key to taking advantage of graph signal processing tools. Most of the conventional algorithms for graph learning require complete data statistics, which might not be available in some scenarios. In this work, we aim to learn a graph from incomplete time-series observations. From another viewpoint, we consider the problem of semi-blind recovery of time-varying graph signals where the underlying graph model is unknown. We propose an algorithm based on the method of block successive upperbound minimization (BSUM), for simultaneous inference of the signal and the graph from incomplete data. Simulation results on synthetic and real time-series demonstrate the performance of the proposed method for graph learning and signal recovery.