Documents
Poster
Poster
JSR-NET: A DEEP NETWORK FOR JOINT SPATIAL-RADON DOMAIN CT RECON- STRUCTION FROM INCOMPLETE DATA
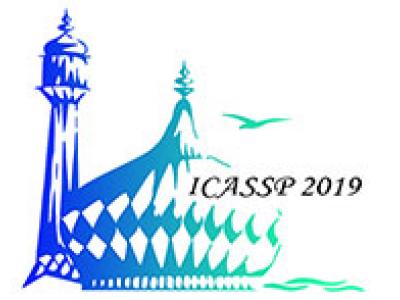
- Citation Author(s):
- Submitted by:
- Haimiao Zhang
- Last updated:
- 10 May 2019 - 3:25am
- Document Type:
- Poster
- Document Year:
- 2019
- Event:
- Presenters:
- Haimiao Zhang
- Paper Code:
- 1283
- Categories:
- Log in to post comments
CT image reconstruction from incomplete data, such as sparse views and limited angle reconstruction, is an important and challenging problem in medical imaging. This work proposes a new deep convolutional neural network (CNN), called JSR-Net, that jointly reconstructs CT images and their associated Radon domain projections. JSR-Net combines the traditional model-based approach with deep architecture design of deep learning. A hybrid loss function is adapted to improve the performance of the JSR-Net making it more effective in protecting important image structures. Numerical experiments demonstrate that JSR-Net outperforms some latest model-based reconstruction methods, as well as a recently proposed deep model.