Documents
Presentation Slides
Presentation Slides
KLM
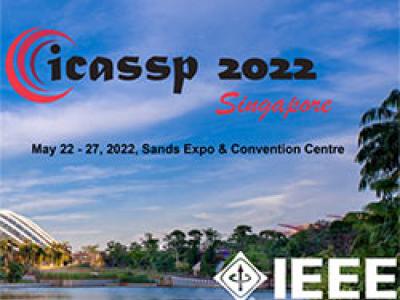
- Citation Author(s):
- Submitted by:
- Petr Tichavsky
- Last updated:
- 6 May 2022 - 3:47am
- Document Type:
- Presentation Slides
- Document Year:
- 2022
- Event:
- Presenters:
- Petr Tichavsky
- Paper Code:
- MLSP-29.4
- Categories:
- Log in to post comments
Structured Tucker tensor decomposition models complete or incomplete multiway data sets (tensors), where the core tensor and the factor matrices can obey different constraints. The model includes block-term decomposition or canonical polyadic decomposition as special cases. We propose a very flexible optimization method for the structured Tucker decomposition problem, based on the second-order Levenberg-Marquardt optimization, using an approximation of the Hessian matrix by the Krylov subspace method. An algorithm with limited sensitivity of the decomposition is included.
The proposed algorithm is shown to perform well in comparison to existing tensor decomposition methods.
slidy22.pdf
