Documents
Presentation Slides
Presentation Slides
The Landscape of Non-convex Quadratic Feasibility
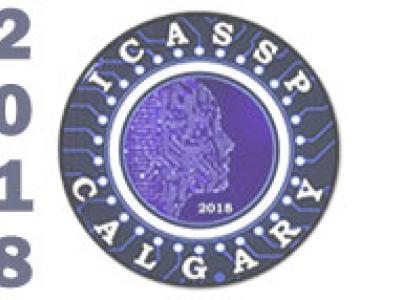
- Citation Author(s):
- Submitted by:
- Amanda Bower
- Last updated:
- 19 April 2018 - 2:10pm
- Document Type:
- Presentation Slides
- Document Year:
- 2018
- Event:
- Presenters:
- Amanda Bower
- Paper Code:
- 4494
- Categories:
- Keywords:
- Log in to post comments
Motivated by applications such as ordinal embedding and collaborative ranking, we formulate homogeneous quadratic feasibility as an unconstrained, non-convex minimization problem. Our work aims to understand the landscape (local minimizers and global minimizers) of the non-convex objective, which corresponds to hinge losses arising from quadratic constraints. Under certain assumptions, we give necessary conditions for non-global, local minimizers of our objective and additionally show that in two dimensions, every local minimizer is a global minimizer. Empirically, we demonstrate that finding feasible points by solving the unconstrained optimization problem with stochastic gradient descent works reliably by utilizing large initializations.
ICASSP_v4.pdf
