Documents
Poster
LANGUAGE MODEL INTEGRATION BASED ON MEMORY CONTROL FOR SEQUENCE TO SEQUENCE SPEECH RECOGNITION
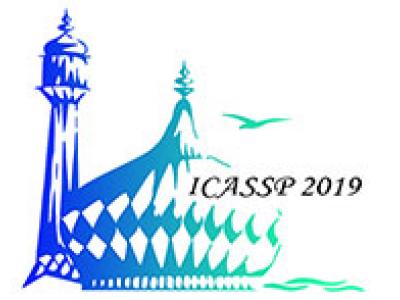
- Citation Author(s):
- Submitted by:
- Jaejin Cho
- Last updated:
- 10 May 2019 - 4:50pm
- Document Type:
- Poster
- Document Year:
- 2019
- Event:
- Presenters:
- Jaejin Cho
- Paper Code:
- SLP-P5.9
- Categories:
- Log in to post comments
In this paper, we explore several new schemes to train a seq2seq model to integrate a pre-trained language model (LM). Our proposed fusion methods focus on the memory cell state and the hidden state in the seq2seq decoder long short-term memory (LSTM), and the memory cell state is updated by the LM unlike the prior studies. This means the memory retained by the main seq2seq would be adjusted by the external LM. These fusion methods have several variants depending on the architecture of this memory cell update and the use of memory cell and hidden states which directly affects the final label inference. We performed the experiments to show the effectiveness of the proposed methods in a mono-lingual ASR setup on the Librispeech corpus and in a transfer learning setup from a multilingual ASR (MLASR) base model to a low-resourced language. In Librispeech, our best model improved WER by 3.7%, 2.4% for test clean, test other relatively to the shallow fusion baseline, with multilevel decoding. In transfer learning from an MLASR base model to the IARPA Babel Swahili model, the best scheme improved the transferred model on eval set by 9.9%, 9.8% in CER, WER relatively to the 2-stage transfer baseline.
Final_final.pdf
