Documents
Poster
Learned Video Compression with Spatial-Temporal Optimization
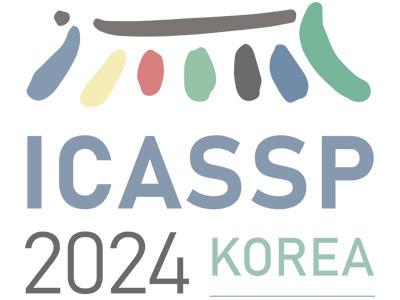
- Citation Author(s):
- Submitted by:
- Yiming Wang
- Last updated:
- 8 April 2024 - 6:14am
- Document Type:
- Poster
- Document Year:
- 2024
- Event:
- Presenters:
- Yiming Wang
- Paper Code:
- IVMSP-P19.7
- Categories:
- Log in to post comments
Previous optical flow based video compression is gradually replaced by unsupervised deformable convolution (DCN) based method. This is mainly due to the fact that the motion vector (MV) estimated by the existing optical flow network
is not accurate and may introduce extra artifacts. However, DCN based method is difficult for training owing to the lack of explicit guidance in the feature space. In this work, we propose a learned video compression with spatial-temporal optimization. Specifically, we first propose the spatial-temporal motion refinement module to improve the accuracy of MV estimated by the optical flow network for prediction. Then, we propose the In-loop filter module to remove compression artifacts and improve the reconstructed frame quality. Finally, comprehensive experimental results demonstrate our proposed method outperforms the recent learned methods on three benchmark datasets. Moreover, our method also beats the H.266/VVC in terms of MS-SSIM metrics.