Documents
Poster
A Learning Approach for Wavelet Design
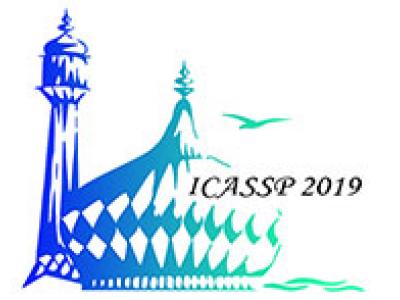
- Citation Author(s):
- Submitted by:
- ABHISHEK KUMAR
- Last updated:
- 10 May 2019 - 2:38am
- Document Type:
- Poster
- Document Year:
- 2019
- Event:
- Presenters:
- Dhruv Jawali, Abhishek Kumar, Chandra Sekhar Seelamantula
- Paper Code:
- 4653
- Categories:
- Log in to post comments
Wavelet analysis and perfect reconstruction filterbanks (PRFBs) are closely related. Desired properties on the wavelet could be translated to equivalent properties on a PRFB. We propose a new learning-based approach towards designing compactly supported orthonormal wavelets with a specified number of vanishing moments. We view PRFBs as a special class of convolutional autoencoders, which places the problem of wavelet/PRFB design within a learning framework. One could then deploy several state-of-the-art deep learning tools to solve the design problem. The PRFBs are learned by minimizing a squared-error loss function using gradient-descent optimization. The model is trained using a dataset containing random samples drawn from the standard normal distribution. We demonstrate that imposing orthonormality and vanishing moment constraints in the learning framework gives rise to filters that generate an orthonormal wavelet basis. We present results for learning PRFBs with filter lengths 2 and 8. As an illustration, we show that the proposed framework is able to learn the Daubechies wavelet with four vanishing moments, as well as wavelets with an arbitrary number of vanishing moments. For all our results, the signal-to-reconstruction error ratio is greater than 200 dB, implying that perfect reconstruction is indeed achieved accurately up to machine precision.