Documents
Presentation Slides
Learning Dynamic Stream Weights for Linear Dynamical Systems using Natural Evolution Strategies
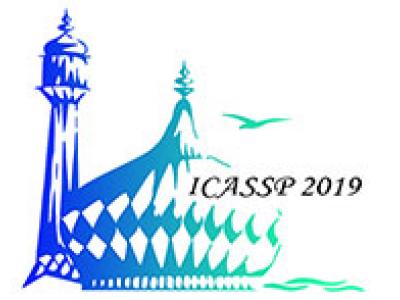
- Citation Author(s):
- Submitted by:
- Christopher Schymura
- Last updated:
- 10 May 2019 - 3:53am
- Document Type:
- Presentation Slides
- Document Year:
- 2019
- Event:
- Presenters:
- Christopher Schymura
- Paper Code:
- 2227
- Categories:
- Log in to post comments
Multimodal data fusion is an important aspect of many object localization and tracking frameworks that rely on sensory observations from different sources. A prominent example is audiovisual speaker localization, where the incorporation of visual information has shown to benefit overall performance, especially in adverse acoustic conditions. Recently, the notion of dynamic stream weights as an efficient data fusion technique has been introduced into this field. Originally proposed in the context of audiovisual automatic speech recognition, dynamic stream weights allow for effective sensory-level data fusion on a per-frame basis, if reliability measures for the individual sensory streams are available. This study proposes a learning framework for dynamic stream weights based on natural evolution strategies, which does not require the explicit computation of oracle information. An experimental evaluation based on recorded audiovisual sequences shows that the proposed approach outperforms conventional methods based on supervised training in terms of localization performance.