Documents
Poster
Learning with Non-Uniform Label Noise: A Cluster-Dependent Weakly Supervised Approach
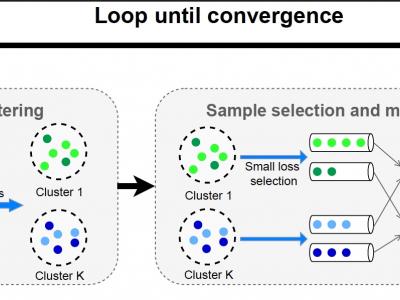
- DOI:
- 10.60864/gnny-nf43
- Citation Author(s):
- Submitted by:
- Mengtian Zhang
- Last updated:
- 6 June 2024 - 10:23am
- Document Type:
- Poster
- Document Year:
- 2024
- Event:
- Presenters:
- Mengtian Zhang
- Paper Code:
- MLSP-P28.9
- Categories:
- Log in to post comments
Learning with noisy labels is a challenging task in machine learning.
Furthermore in reality, label noise can be highly non-uniform
in feature space, e.g. with higher error rate for more difficult samples.
Some recent works consider instance-dependent label noise
but they require additional information such as some cleanly labeled
data and confidence scores, which are usually unavailable or costly
to obtain. In this paper, we consider learning with non-uniform label
noise that requires no such additional information. Inspired by
stratified sampling, we propose a cluster-dependent sample selection
algorithm followed by a contrastive training mechanism based
on the cluster-dependent label noise. Despite its simplicity, the proposed
method can distinguish clean data from the corrupt ones more
precisely and achieve state-of-the-art performance on most image
classification benchmarks, especially when the number of training
samples is small and the noise rate is high.