Documents
Presentation Slides
Learning Robust Features for 3D Object Pose Estimation
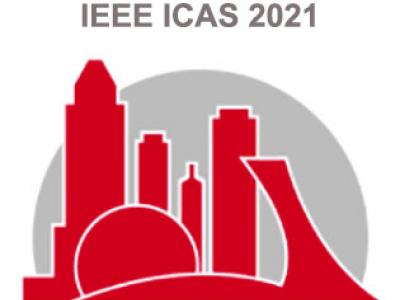
- Citation Author(s):
- Submitted by:
- Christos Papaio...
- Last updated:
- 29 October 2021 - 6:24am
- Document Type:
- Presentation Slides
- Document Year:
- 2021
- Event:
- Presenters:
- Ioannis Pitas
- Categories:
- Log in to post comments
Object pose estimation remains an open and important task for autonomous systems, allowing them to perceive and interact with the surrounding environment. To this end, this paper proposes a 3D object pose estimation method that is suitable for execution on embedded systems. Specifically, a novel multi-task objective function is proposed, in order to train a Convolutional Neural Network (CNN) to extract pose-related features from RGB images, which are subsequently utilized in a Nearest-Neighbor (NN) search-based post-processing step to obtain the final 3D object poses. By utilizing a symmetry-aware term and unit quaternions in the proposed objective function, our method yielded more robust and discriminative features, thus, increasing 3D object pose estimation accuracy when compared to state-of-the-art. In addition, the employed feature extraction network utilizes a lightweight CNN architecture, allowing execution on hardware with limited computational capabilities. Finally, we demonstrate that the proposed method is also able to successfully generalize to previously unseen objects, without the need for extra training.