Documents
Poster
LEARNING SPECTRAL CANONICAL F-CORRELATION REPRESENTATION FOR FACE SUPER-RESOLUTION
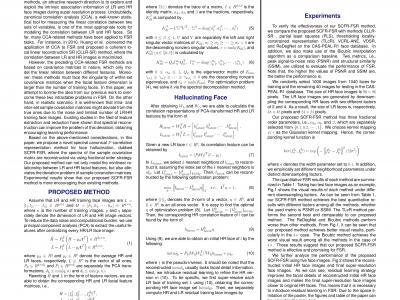
- DOI:
- 10.60864/whw9-y626
- Citation Author(s):
- Submitted by:
- MingZhi Hao
- Last updated:
- 14 July 2024 - 5:31am
- Document Type:
- Poster
- Document Year:
- 2024
- Event:
- Presenters:
- Yun-Hao Yuan,MingZhi Hao
- Paper Code:
- 9615
- Categories:
- Log in to post comments
Face super-resolution (FSR) is a powerful technique for restoring high-resolution face images from the captured low-resolution ones with the assistance of prior information. Existing FSR methods based on explicit or implicit covariance matrices are difficult to reveal complex nonlinear relationships between features, as conventional covariance computation is essentially a linear operation process. Besides, the limited number of training samples and noise disturbance lead to the deviation of sample covariance matrices. To solve these issues, we propose a novel FSR method via using spectral canonical $\mathcal{F}$-correlation representation. The proposed method first defines intra-resolution and inter-resolution covariation matrices by considering the nonlinear relationship between different features, and then uses the fractional order idea to rebuild covariation matrices. The qualitative and quantitative results have validated the superiority of the proposed method.