Documents
Presentation Slides
The Limitation and Practical Acceleration of Stochastic Gradient Algorithms in Inverse Problems
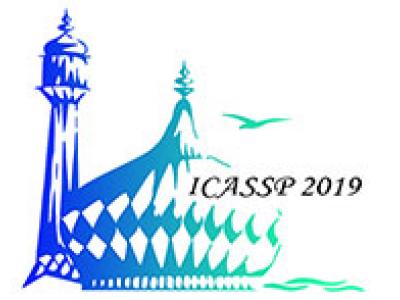
- Citation Author(s):
- Submitted by:
- Junqi Tang
- Last updated:
- 14 May 2019 - 6:05pm
- Document Type:
- Presentation Slides
- Document Year:
- 2019
- Event:
- Presenters:
- Junqi Tang
- Paper Code:
- CI-L1.4
- Categories:
- Log in to post comments
In this work we investigate the practicability of stochastic gradient descent and recently introduced variants with variance-reduction techniques in imaging inverse problems, such as space-varying image deblurring. Such algorithms have been shown in machine learning literature to have optimal complexities in theory, and provide great improvement empirically over the full gradient methods. Surprisingly, in some tasks such as image deblurring, many of such methods fail to converge faster than the accelerated full gradient method (FISTA), even in terms of epoch counts. We investigate this phenomenon and propose a theory-inspired mechanism to characterize whether a given inverse problem should be preferred to be solved by stochastic optimization technique with a known sampling pattern. Furthermore, to overcome another key bottleneck of stochastic optimization which is the heavy computation of proximal operators while maintaining fast convergence, we propose an accelerated primal-dual SGD algorithm and demonstrate the effectiveness of our approach in image deblurring experiments.