Documents
Presentation Slides
Presentation Slides
Low-complexity Recurrent Neural Network-based Polar Decoder with Weight Quantization Mechanism
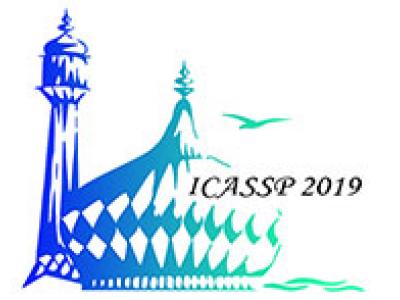
- Citation Author(s):
- Submitted by:
- Chieh-Fang Teng
- Last updated:
- 7 May 2019 - 8:34pm
- Document Type:
- Presentation Slides
- Document Year:
- 2019
- Event:
- Presenters:
- Chieh-Fang Teng
- Paper Code:
- DISPS-L2.1
- Categories:
- Log in to post comments
Polar codes have drawn much attention and been adopted in 5G New Radio (NR) due to their capacity-achieving performance. Recently, as the emerging deep learning (DL) technique has breakthrough achievements in many fields, neural network decoder was proposed to obtain faster convergence and better performance than belief propagation (BP) decoding. However, neural networks are memory-intensive and hinder the deployment of DL in communication systems. In this work, a low-complexity recurrent neural network (RNN) polar decoder with codebook-based weight quantization is proposed. Our test results show that we can effectively reduce the memory overhead by 98% and alleviate computational complexity with slight performance loss.