Documents
Presentation Slides
Presentation Slides
Low-cost Measurement of Industrial Shock Signals via Deep Learning Calibration
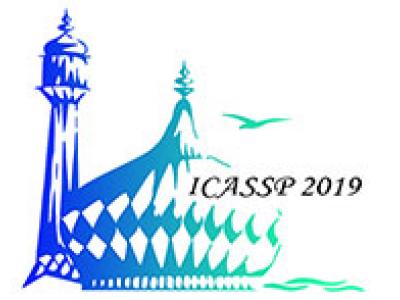
- Citation Author(s):
- Submitted by:
- Ze Ji
- Last updated:
- 9 May 2019 - 9:30am
- Document Type:
- Presentation Slides
- Document Year:
- 2019
- Event:
- Presenters:
- Ze Ji
- Paper Code:
- MLSP-L5.4
- Categories:
- Log in to post comments
Special high-end sensors with expensive hardware are usually needed to measure shock signals with high accuracy. In this paper, we show that cheap low-end sensors calibrated by deep neural networks are also capable to measure high-g shocks accurately. Firstly we perform drop shock tests to collect a dataset of shock signals measured by sensors of different fidelity. Secondly, we propose a novel network to effectively learn both the signal peak and overall shape. The results show that the proposed network is capable to map low-end shock signals to its high-end counterparts with satisfactory accuracy. To the best of our knowledge, this is the first work to apply deep learning techniques to calibrate shock sensors.