Documents
Poster
Poster
Lowering Dynamic Power of a Stream-based CNN Hardware Accelerator
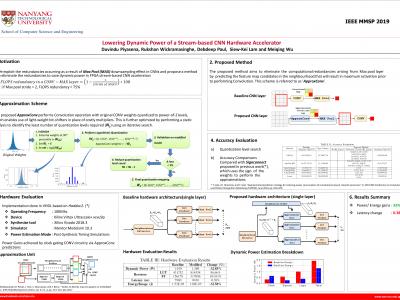
- Citation Author(s):
- Submitted by:
- Duvindu Piyasena
- Last updated:
- 26 September 2019 - 8:30pm
- Document Type:
- Poster
- Document Year:
- 2019
- Event:
- Paper Code:
- 6112101
- Categories:
- Keywords:
- Log in to post comments
Custom hardware accelerators of Convolutional Neural Networks (CNN) provide a promising solution to meet real-time constraints for a wide range of applications on low-cost embedded devices. In this work, we aim to lower the dynamic power of a stream-based CNN hardware accelerator by reducing the computational redundancies in the CNN layers. In particular, we investigate the redundancies due to the downsampling effect of max pooling layers which are prevalent in state-of-the-art CNNs, and propose an approximation method to reduce the overall computations. The experimental results show that the proposed method leads to lower dynamic power without sacrificing accuracy.