Documents
Poster
A Machine-Learning Model for Detecting Depression, Anxiety, and Stress from Speech
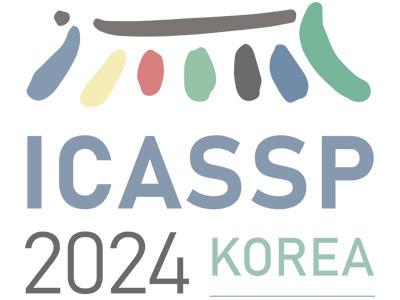
- DOI:
- 10.60864/enyh-qr76
- Citation Author(s):
- Submitted by:
- Mashrura Tasnim
- Last updated:
- 6 June 2024 - 10:28am
- Document Type:
- Poster
- Document Year:
- 2024
- Event:
- Presenters:
- Mashrura Tasnim
- Paper Code:
- 8111
- Categories:
- Log in to post comments
Predicting mental health conditions from speech has been widely explored in recent years. Most studies rely on a single sample from each subject to detect indicators of a particular disorder. These studies ignore two important facts: certain mental disorders tend to co-exist, and their severity tends to vary over time. This work introduces a longitudinal dataset labeled with depression, anxiety, and stress scores using the DASS-21 self-report questionnaire, and describes a machine-learning pipeline to determine the severity of the three mental disorders using acoustic features extracted from speech samples of this dataset. Our initial findings suggest that healthy participants adhere more to the study procedure than participants who exhibit indicators of depression, anxiety, and stress and demonstrate that a one-dimensional convolutional neural network, trained on VGG-19 features, predicts the severity of depression, anxiety, and stress with high accuracy.