Documents
Poster
Matching Pursuit Based on Kernel Non-second Order Minimization
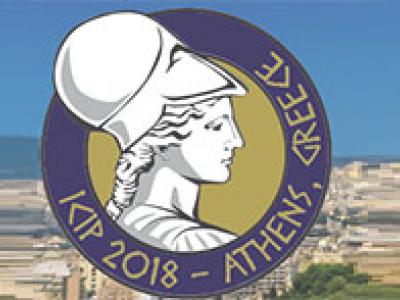
- Citation Author(s):
- Submitted by:
- Miaohua Zhang
- Last updated:
- 4 October 2018 - 7:56pm
- Document Type:
- Poster
- Document Year:
- 2018
- Event:
- Presenters:
- Miaohua Zhang
- Paper Code:
- #3017
- Categories:
- Keywords:
- Log in to post comments
The orthogonal matching pursuit (OMP) is an important sparse approximation algorithm to recover sparse signals from compressed measurements. However, most MP algorithms are based on the mean square error(MSE) to minimize the recovery error, which is suboptimal when there are outliers. In this paper, we present a new robust OMP algorithm based on kernel non-second order statistics (KNS-OMP), which not only takes advantages of the outlier resistance ability of correntropy but also further extends the second order statistics based correntropy to a non-second order similarity measurement to improve its robustness. The resulted framework is more accurate than the second order ones in reducing the effect of outliers. Experimental results on synthetic and real data show that the proposed method achieves better performances compared with existing methods.