Documents
Poster
A Minimally Supervised Approach for Medical Image Quality Assessment in Domain Shift Settings
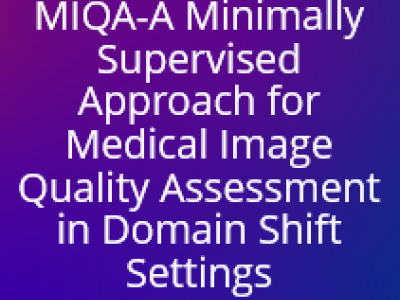
- Citation Author(s):
- Submitted by:
- Huijuan Yang
- Last updated:
- 12 May 2022 - 11:03pm
- Document Type:
- Poster
- Document Year:
- 2022
- Event:
- Presenters:
- Huijuan Yang
- Paper Code:
- BIO-P1.6
- Categories:
- Log in to post comments
Accurate disease diagnosis requires objective assessment of clinical image quality. Automated image quality assessment (IQA) could enhance screening and diagnosis workflows. However, development of generalizable quality assessment tools requires large labeled clinical image datasets from different sites. Obtaining these datasets is often infeasible; and quality indicators may vary with acquisition settings due to domain shift. We introduce a minimally-supervised
image quality assessment (MIQA) approach that can learn effectively with small datasets and limited labels in class-imbalanced domain shift scenarios. We formulate the IQA task as an anomaly detection problem, and use a small number of target domain images to identify a compact subset of source domain data for better representation of acceptable quality features. For this compact source domain dataset, we extract features with a pre-trained CNN, perform adaptive
feature selection, and develop a one-class classifier to detect poor quality images. We evaluate our approach on two ophthalmology datasets, and show substantial AUC gains and improved cross-site generalizability over competitive baselines. Our approach has implications for improved image quality audit in many clinical settings.
8754-2.pdf
