Documents
Poster
MMCosine: Multi-Modal Cosine Loss Towards Balanced Audio-Visual Fine-Grained Learning
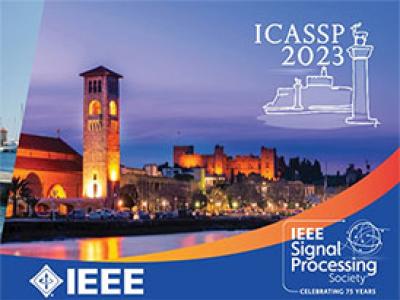
- DOI:
- 10.60864/tsem-h053
- Citation Author(s):
- Submitted by:
- Ruize Xu
- Last updated:
- 17 November 2023 - 12:07pm
- Document Type:
- Poster
- Document Year:
- 2023
- Event:
- Presenters:
- Ruize Xu
- Paper Code:
- IVMSP-P20.6
- Categories:
- Log in to post comments
Audio-visual learning helps to comprehensively understand the world by fusing practical information from multiple modalities. However, recent studies show that the imbalanced optimization of uni-modal encoders in a joint-learning model is a bottleneck to enhancing the model`s performance. We further find that the up-to-date imbalance-mitigating methods fail on some audio-visual fine-grained tasks, which have a higher demand for distinguishable feature distribution.
Fueled by the success of cosine loss that builds hyperspherical feature spaces and achieves lower intra-class angular variability, this paper proposes Multi-Modal Cosine loss, MMCosine. It performs a modality-wise L2 normalization to features and weights towards balanced and better multi-modal fine-grained learning. We demonstrate that our method can alleviate the imbalanced optimization from the perspective of weight norm and fully exploit the discriminability of the cosine metric.
Extensive experiments prove the effectiveness of our method and the versatility with advanced multi-modal fusion strategies and up-to-date imbalance-mitigating methods.