Documents
Poster
MULTI-DIRECTIONAL CONVOLUTION NETWORKS WITH SPATIAL-TEMPORAL FEATURE PYRAMID MODULE FOR ACTION RECOGNITION
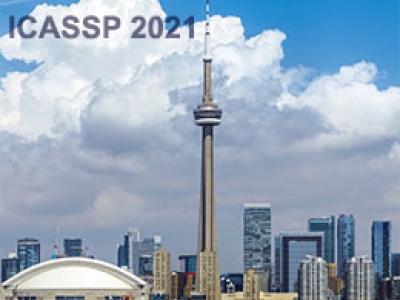
- Citation Author(s):
- Submitted by:
- Bohong Yang
- Last updated:
- 1 July 2021 - 8:40am
- Document Type:
- Poster
- Document Year:
- 2021
- Event:
- Presenters:
- Bohong Yang
- Paper Code:
- IVMSP-33.2
- Categories:
- Log in to post comments
Recent attempts show that factorizing 3D convolutional filters into separate spatial and temporal components brings impressive improvement in action recognition. However, traditional temporal convolution operating along the temporal dimension will aggregate unrelated features, since the feature maps of fast-moving objects have shifted spatial positions. In this paper, we propose a novel and effective Multi-Directional convolution (MDConv), which extracts features along different spatial-temporal orientations. Especially, MDConv has the same FLOPs and parameters as the traditional 1D temporal convolution. Also, we propose the Spatial-Temporal Feature Pyramid Module (STFPM) to fuse spatial semantics in different scales in a light-weight way. Our extensive experiments show that the models which integrate with MDConv achieve better accuracy on several large-scale action recognition benchmarks such as Kinetics, Something-Something V1&V2 and AVA datasets.
poster-4.pdf
