Documents
Poster
MULTI-MODALITY ACTION RECOGNITION BASED ON DUAL FEATURE SHIFT IN VEHICLE CABIN MONITORING
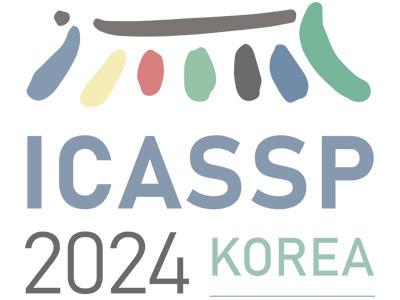
- DOI:
- 10.60864/pcgd-gf04
- Citation Author(s):
- Submitted by:
- Yiming Li
- Last updated:
- 6 June 2024 - 10:21am
- Document Type:
- Poster
- Document Year:
- 2024
- Event:
- Presenters:
- YIMING LI
- Paper Code:
- MLSP-P35.8
- Categories:
- Log in to post comments
Driver Action Recognition (DAR) is crucial in vehicle cabin monitoring systems. In real-world applications, it is common for vehicle cabins to be equipped with cameras featuring different modalities. However, multi-modality fusion strategies for the DAR task within car cabins have rarely been studied. In this paper, we propose a novel yet efficient multi-modality driver action recognition method based on dual feature shift, named DFS. DFS first integrates complementary features across modalities by performing modality feature interaction. Meanwhile, DFS achieves the neighbour feature propagation within single modalities, by feature shifting among temporal frames. To learn common patterns and improve model efficiency, DFS shares feature extracting stages among multiple modalities. Extensive experiments have been carried out to verify the effectiveness of the proposed DFS model on the Drive&Act dataset. The results demonstrate that DFS achieves good performance and improves the efficiency of multi-modality driver action recognition.