Documents
Presentation Slides
Multi-View Self-Attention based Transformer for Speaker Recognition
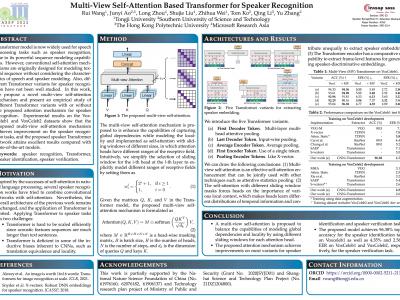
- Citation Author(s):
- Submitted by:
- Rui Wang
- Last updated:
- 5 May 2022 - 3:54am
- Document Type:
- Presentation Slides
- Document Year:
- 2022
- Event:
- Presenters:
- Rui Wang
- Paper Code:
- SPE-25.4
- Categories:
- Log in to post comments
Initially developed for natural language processing (NLP), Transformer model is now widely used for speech processing tasks such as speaker recognition, due to its powerful sequence modeling capabilities. However, conventional self-attention mechanisms are originally designed for modeling textual sequence without considering the characteristics of speech and speaker modeling. Besides, different Transformer variants for speaker recognition have not been well studied. In this work, we propose a novel multi-view self-attention mechanism and present an empirical study of different Transformer variants with or without the proposed attention mechanism for speaker recognition. Specifically, to balance the capabilities of capturing global dependencies and modeling the locality, we propose a multi-view self-attention mechanism for speaker Transformer, in which different attention heads can attend to different ranges of the receptive field. Furthermore, we introduce and compare five Transformer variants with different network architectures, embedding locations, and pooling methods to learn speaker embeddings. Experimental results on the VoxCeleb1 and VoxCeleb2 datasets show that the proposed multi-view self-attention mechanism achieves improvement in the performance of speaker recognition, and the proposed speaker Transformer network attains excellent results compared with state-of-the-art models.