Documents
Poster
MULTIMODAL EMOTION RECOGNITION BASED ON DEEP TEMPORAL FEATURES USING CROSS-MODAL TRANSFORMER AND SELF-ATTENTION
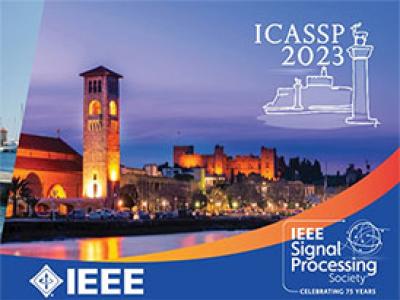
- Citation Author(s):
- Submitted by:
- BUBAI MAJI
- Last updated:
- 27 May 2023 - 7:04am
- Document Type:
- Poster
- Document Year:
- 2023
- Event:
- Presenters:
- Monorama Swain
- Categories:
- Log in to post comments
Multimodal speech emotion recognition (MSER) is an emerging and challenging field of research due to its more robust characteristics than unimodal. However, in multimodal approaches, the interactive relations for model building using different modalities of speech representations for emotion recognition have not been well investigated yet. To address this issue, we introduce a new approach to capturing the deep temporal features of audio and text. The audio features are learned with a convolution neural network (CNN) and a Bi-directional Gated Recurrent Unit (Bi-GRU) network. The textual features are represented by GloVe word embedding along with Bi-GRU. A cross-modal transformers block is designed for multimodal learning to capture better inter- and intra-interactions and temporal information between the audio and textual features. Further, a self-attention (SA) network is employed to select more important emotional information from the fused multimodal features. We evaluate the proposed method on the IEMOCAP dataset on four emotion classes (i.e., angry, neutral, sad, and happy). The proposed method performs significantly better than the most recent state-of-the-art MSER methods.