Documents
Poster
MULTITASK LEARNING FOR FRAME-LEVEL INSTRUMENT RECOGNITION
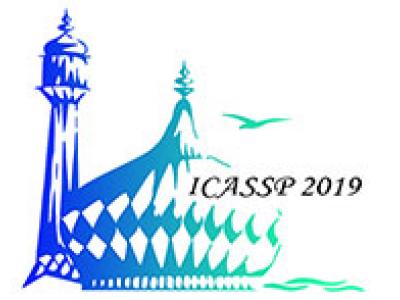
- Citation Author(s):
- Submitted by:
- Yun-Ning Hung
- Last updated:
- 7 May 2019 - 6:02pm
- Document Type:
- Poster
- Document Year:
- 2019
- Event:
- Presenters:
- Yun-Ning Hung
- Paper Code:
- 2803
- Categories:
- Log in to post comments
For many music analysis problems, we need to know the presence
of instruments for each time frame in a multi-instrument
musical piece. However, such a frame-level instrument recognition
task remains difficult, mainly due to the lack of labeled
datasets. To address this issue, we present in this paper a
large-scale dataset that contains synthetic polyphonic music
with frame-level pitch and instrument labels. Moreover, we
propose a simple yet novel network architecture to jointly predict
the pitch and instrument for each frame. With this multitask
learning method, the pitch information can be leveraged
to predict the instruments, and also the other way around.
And, by using the so-called pianoroll representation of music
as the main target output of the model, our model also predicts
the instruments that play each individual note event. We
validate the effectiveness of the proposed method for framelevel
instrument recognition by comparing it with its singletask
ablated versions and three state-of-the-art methods. We
also demonstrate the result of the proposed method for multipitch
streaming with real-world music.