Documents
Presentation Slides
Multiview Long-Short Spatial Contrastive Learning for 3D Medical Image Analysis
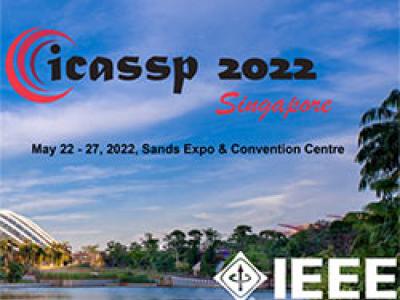
- Citation Author(s):
- Submitted by:
- Gongpeng Cao
- Last updated:
- 15 May 2022 - 11:29am
- Document Type:
- Presentation Slides
- Document Year:
- 2022
- Event:
- Presenters:
- Gongpeng Cao
- Paper Code:
- BIO-5.6
- Categories:
- Log in to post comments
The success of supervised deep learning heavily depends on large labeled datasets whose construction is often challenging in medical image analysis. Contrastive learning, a variant of self-supervised learning, is a potential solution to alleviate the strong demand for data annotation. In this work, we extend the contrastive learning framework to 3D volumetric medical imaging. Specifically, we propose (1) multiview contrasting strategy to maximize the mutual information between three views of 3D image to learn global representations and (2) long-short spatial contrasting strategy to learn local representations by matching a short spatial clip to a long spatial clip in the latent space. To combine these two strategies, we propose multiview long-short spatial contrastive learning (MLSSCL) framework, which can effectively learn generic 3D representations. Our extensive experiments on two brain Magnetic Resonance Imaging (MRI) datasets demonstrate that MLSSCL significantly outperforms learning from scratch and other self-supervised learning methods on both classification and segmentation tasks.