Documents
Poster
Network Adaptation Strategies for Learning New Classes without Forgetting the Original Ones
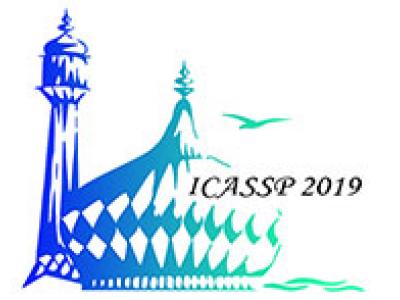
- Citation Author(s):
- Submitted by:
- Hagai Taitelbaum
- Last updated:
- 15 May 2019 - 7:51am
- Document Type:
- Poster
- Document Year:
- 2019
- Event:
- Presenters:
- Hagai Taitelbaum
- Paper Code:
- MLSP-P13.5
- Categories:
- Log in to post comments
We address the problem of adding new classes to an existing classifier without hurting the original classes, when no access is allowed to any sample from the original classes. This problem arises frequently since models are often shared without their training data, due to privacy and data ownership concerns. We propose an easy-to-use approach that modifies the original classifier by retraining a suitable subset of layers using a linearly-tuned, knowledge-distillation regularization. The set of layers that is tuned depends on the number of new added classes and the number of original classes.We evaluate the proposed method on two standard datasets, first in a language-identification task, then in an image classification setup. In both cases, the method achieves classification accuracy that is almost as good as that obtained by a system trained using unrestricted samples from both the original and new classes.