Documents
Poster
A NOVEL MONOCULAR DISPARITY ESTIMATION NETWORK WITH DOMAIN TRANSFORMATION AND AMBIGUITY LEARNING
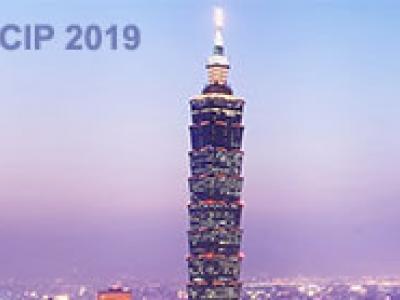
- Citation Author(s):
- Submitted by:
- Juan Gonzalez
- Last updated:
- 19 September 2019 - 8:16am
- Document Type:
- Poster
- Event:
- Presenters:
- Juan Luis Gonzalez
- Paper Code:
- 1748
- Categories:
- Log in to post comments
Convolutional neural networks (CNN) have shown state-of-the-art results for low-level computer vision problems such as stereo and monocular disparity estimations, but still, have much room to further improve their performance in terms of accuracy, numbers of parameters, etc. Recent works have uncovered the advantages of using an unsupervised scheme to train CNN’s to estimate monocular disparity, where only the relatively-easy-to-obtain stereo images are needed for training. We propose a novel encoder-decoder architecture that outperforms previous unsupervised monocular depth estimation networks by (i) taking into account ambiguities, (ii) efficient fusion between encoder and decoder features with rectangular convolutions and (iii) domain transformations between encoder and decoder. Our architecture outperforms the Monodepth baseline in all metrics, even with a considerable reduction of parameters. Furthermore, our architecture is capable of estimating a full disparity map in a single forward pass, whereas the baseline requires two passes. We perform extensive experiments to verify the effectiveness of our method on the KITTI dataset.