Documents
Poster
nRPN: Hard Example Learning for Region Proposal Networks
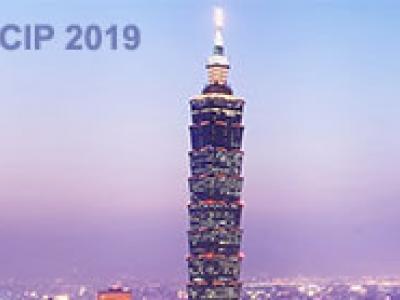
- Citation Author(s):
- Submitted by:
- Myeong Ah Cho
- Last updated:
- 16 September 2019 - 5:03am
- Document Type:
- Poster
- Document Year:
- 2019
- Event:
- Presenters:
- MyeongAh Cho
- Paper Code:
- WP.PB.7
- Categories:
- Log in to post comments
The region proposal task is generating a set of candidate regions that contain an object. In this task, it is most important to propose as many candidates of ground-truth in a fixed number of proposals. However, in an image, there are too small number of hard negative examples compared to the vast number of easy negatives, so the region proposal networks struggle to train hard negatives. Because of these problem, network tends to propose hard negatives as the candidates and fails to propose the ground-truth, which leads poor performance.
In this paper, we propose Negative Region Proposal Network(nRPN) to improve Region Proposal Network(RPN). nRPN learns from false positives of RPN and provides hard negative examples to RPN. Our proposed nRPN leads to reduce false positives and better performance of RPN. Also RPN which trained with nRPN achieves performance improvement on PASCAL VOC 2007 dataset.