Documents
Presentation Slides
Online Learning for Indoor Asset Detection
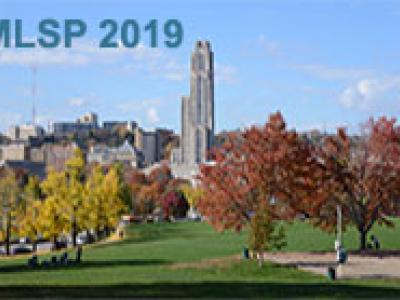
- Citation Author(s):
- Submitted by:
- Adith Balamurugan
- Last updated:
- 14 October 2019 - 8:33pm
- Document Type:
- Presentation Slides
- Document Year:
- 2019
- Event:
- Presenters:
- Adith Balamurugan
- Paper Code:
- 48
- Categories:
- Log in to post comments
Building floor plans with locations of safety, security, and energy assets such as IoT sensors, fire alarms, etc. are vital for climate control, emergency response, safety, and maintenance of building infrastructure. Existing approaches to building survey are tedious, error prone, and involve an operator with a clipboard and pen, enumerating and localizing assets in each room. We propose an interactive method for a human operator to use an app on a smartphone, which can detect, classify, and localize assets of interest, to expedite such a task. We must overcome the fact that appearances of a single type of asset, e.g. power outlet, vary greatly from building to building or even from room to room. We propose an online "one-shot learning" approach using a Neural Turing Machine (NTM) architecture with augmented memory capacity, which allows us to rapidly incorporate new data into our model, improving prediction accuracy after only a few examples, without compromising its ability to remember previously learned data. This approach reduces the training time needed to update the model between building survey sessions by up to a factor of 10. Experiments show that our proposed method outperforms the prediction accuracy attained by using more traditional, batch processing deep learning methods where new data is combined with all old data to train the model. The advantage is especially pronounced for assets in never-before-seen buildings.