Documents
Presentation Slides
Open-Set deepfake detection to fight the unknown
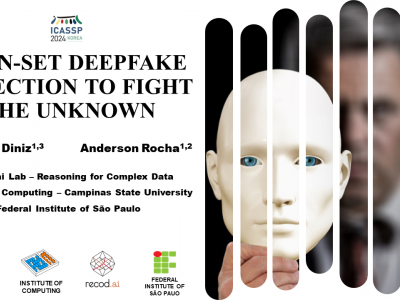
- DOI:
- 10.60864/s5rv-nf63
- Citation Author(s):
- Submitted by:
- Michael Diniz
- Last updated:
- 6 June 2024 - 10:50am
- Document Type:
- Presentation Slides
- Document Year:
- 2024
- Event:
- Presenters:
- Michael Macedo Diniz
- Paper Code:
- 4642
- Categories:
- Log in to post comments
In this paper, we design a new open-set method to detect deepfakes that does not assume information about the techniques behind the deepfakes generation. Contrary to existing methods, which build upon known telltales left by the deepfake creation process, we assume no prior knowledge about the sample generation, thus presenting a method for blind deepfake detection, a necessary step toward true generalization. Our methodology relies upon unsupervised learning, open-set formulations for each discovered group and, finally, relevance tests through extreme-value theory and isolation forest formulations. The results indicate that the proposed open-set technique is competitive with state-of-the-art closed-set deepfake detection methods. As a notable outcome, we achieved an AUC = 0.807, which is 5.57% higher than the baseline architecture trained using a closed-set approach. Finally, we believe our efforts herein are just a first attempt tackling this difficult problem and discuss some additional improvements for practical deployment of such systems.