Documents
Poster
OPEN-SET RECOGNITION FOR FACIAL-EXPRESSION RECOGNITION
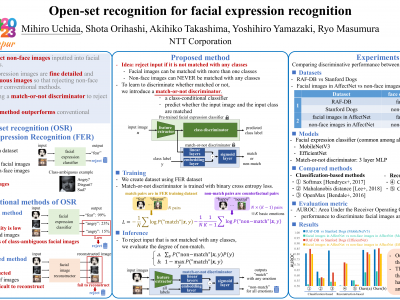
- DOI:
- 10.60864/94z2-5a47
- Citation Author(s):
- Submitted by:
- Mihiro Uchida
- Last updated:
- 17 November 2023 - 12:05pm
- Document Type:
- Poster
- Document Year:
- 2023
- Event:
- Presenters:
- Mihiro Uchida
- Paper Code:
- MP2.PB1
- Categories:
- Keywords:
- Log in to post comments
We address distinguishing whether an input is a facial image by learning only a facial-expression recognition (FER) dataset.
To avoid misclassification in FER, it is necessary to distinguish whether the input is a facial image. Unfortunately, collecting exhaustive nonface images is costly. Therefore, distinguishing whether the input is a facial image by learning only an FER dataset is important. A representative method for this task is learning reconstruction of only facial images and determining high-error samples between input images and reconstructed images as non-face images.
However, reconstruction is difficult on facial images because such images contain detailed features. Our key idea to tackle the task without reconstruction is assuming that facial images will match several emotions, whereas non-face images will not match any emotion.
Therefore, we propose a method for training a discriminator that determines whether the inputs and emotions match using counterfactual pairs in an FER dataset. A metric for the task is then obtained by taking into account each emotion in the posterior probability that inputs and emotions match, estimated by the discriminator.
Experiments on the RAF-DB dataset vs. the Stanford Dogs dataset and AffectNet datasets showed the effectiveness of our method.