Documents
Poster & Presentation Slides
ORCA-PARTY: An Automtatic Killer Whale Sound Type Separation Toolkit Using Deep Learning
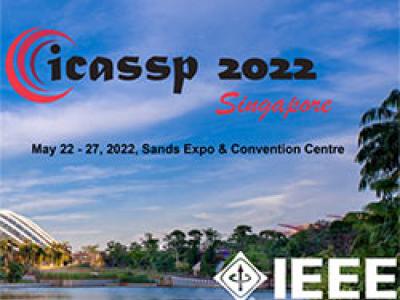
- Citation Author(s):
- Submitted by:
- Christian Bergler
- Last updated:
- 5 May 2022 - 3:53am
- Document Type:
- Poster & Presentation Slides
- Document Year:
- 2022
- Event:
- Presenters:
- Christian Bergler
- Categories:
- Log in to post comments
Data-driven and machine-based analysis of massive bioacoustic data collections, in particular acoustic regions containing a substantial number of vocalizations events, is essential and extremely valuable to identify recurring vocal paradigms. However, these acoustic sections are usually characterized by a strong incidence of overlapping vocalization events, a major problem severely affecting subsequent human-/machine-based analysis and interpretation. Robust machine-driven signal separation of species-specific call types is extremely challenging due to missing ground truth data, speaker/source-relevant information, limited knowledge about inter- and intra-call type variations, next to diverse recording conditions. The current study is the first introducing a fully-automated deep signal separation approach for overlapping orca vocalizations, addressing all of the previously mentioned challenges, together with one of the largest bioacoustic data archives recorded on killer whales (Orcinus Orca). Incorporating ORCA-PARTY as additional data enhancement step for downstream call type classification demonstrated to be extremely valuable. Besides the proof of cross-domain applicability and consistently promising results on non-overlapping signals, significant improvements were achieved when processing acoustic orca segments comprising a multitude of vocal activities. Apart from auspicious visual inspections, a final numerical evaluation on an unseen dataset proved that about 30 % more known sound patterns could be identified.