Documents
Poster
Overcoming Measurement Inconsistency in Deep Learning for Linear Inverse Problems: Applications in Medical Imaging
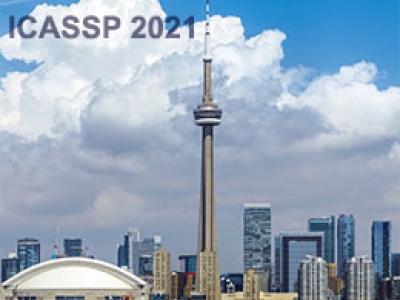
- Citation Author(s):
- Submitted by:
- Marija Vella
- Last updated:
- 1 July 2021 - 10:49am
- Document Type:
- Poster
- Document Year:
- 2021
- Event:
- Presenters:
- Marija Vella
- Paper Code:
- SS-2.6
- Categories:
- Log in to post comments
The remarkable performance of deep neural networks (DNNs) currently makes them the method of choice for solving linear inverse problems. They have been applied to super-resolve and restore images, as well as to reconstruct MR and CT images. In these applications, DNNs invert a forward operator by finding, via training data, a map between the measurements and the input images. It is then expected that the map is still valid for the test data. This framework, however, introduces measurement inconsistency during testing. We show that such inconsistency, which can be critical in domains like medical imaging or defense, is intimately related to the generalization error. We then propose a framework that post-processes the output of DNNs with an optimization algorithm that enforces measurement consistency. Experiments on MR images show that enforcing measurement consistency via our method can lead to large gains in reconstruction performance