Documents
Poster
Poster
Pairwise Approximate K-SVD
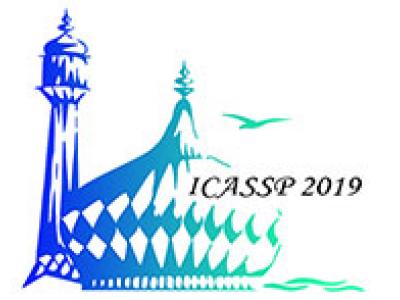
- Citation Author(s):
- Submitted by:
- Paul Irofti
- Last updated:
- 10 May 2019 - 3:58am
- Document Type:
- Poster
- Document Year:
- 2019
- Event:
- Presenters:
- Paul Irofti
- Paper Code:
- MLSP-P14
- Categories:
- Log in to post comments
Pairwise, or separable, dictionaries are suited for the sparse representation of 2D signals in their original form, without vectorization. They are equivalent with enforcing a Kronecker structure on a standard dictionary for 1D signals. We present a dictionary learning algorithm, in the coordinate descent style of Approximate K-SVD, for such dictionaries. The algorithm has the benefit of extremely low complexity, clearly lower than that of existing algorithms. Experimental evidence shows that the performance of the proposed algorithm is comparable to that of standard (unstructured) AK-SVD with the same number of atoms.