Documents
Presentation Slides
Pairwise Learning using Multi-lingual Bottleneck Features for Low-resource Query-by-example Spoken Term Detection
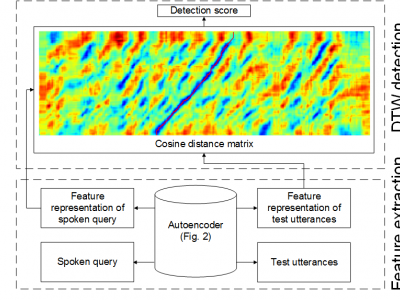
- Citation Author(s):
- Submitted by:
- Yougen Yuan
- Last updated:
- 7 March 2017 - 11:01pm
- Document Type:
- Presentation Slides
- Document Year:
- 2017
- Event:
- Presenters:
- Yougen Yuan
- Paper Code:
- 1280
- Categories:
- Log in to post comments
We propose to use a feature representation obtained by pairwise learning in a low-resource language for query-by-example spoken term detection (QbE-STD). We assume that word pairs identified by humans are available in the low-resource target language. The word pairs are parameterized by a multi-lingual bottleneck feature (BNF) extractor that is trained using transcribed data in high-resource languages. The multi-lingual BNFs of the word pairs are used as an initial feature representation to train an autoencoder (AE). We extract features from an internal hidden layer of the pairwise trained AE to perform acoustic pattern matching for QbE-STD. Our experiments on the TIMIT and Switchboard corpora show that the pairwise learning brings 7.61% and 8.75% relative improvements in mean average precision (MAP) respectively over the initial feature representation.